Decoding Nvidia: Chips, Code, and Innovation
Download MP3Dalton (00:01)
Welcome to VentureStep podcast where we will, we are still figuring out what we're learning. I haven't figured that one out this week. I'll probably get back to you next week, but today, fortunately enough, I do know what we'll be talking about. We're going to be talking about three gentlemen in the early nineties who wanted to found a company that would better serve an up and growing industry related to computers and
what they wanted to do was create a product that would serve as an integral part of computers for computer graphics. And that company was founded in a Denny's, I guess. I didn't know that, but the company that I'm talking, I want to talk about today has their hands in many lucrative cookie jars, including AI development, AI.
GPUs that are used or just API AI chips or they have fabricators they have a full vertical integration and the company that we're going to be talking about today is Nvidia and the objective today is just just to learn about Nvidia there's a lot of crazy lore related to Nvidia maybe because of the gamers I mean game gamers the gamer
industry or gaming industry definitely has some lore aspects. So a lot of people will document things that are going on. But today we'll just turn, you know, we want to just learn about Nvidia and their history, their products, what they do, some of their core competencies. And then, you know, we'll discuss like what a CPU is or GPU is or like architecture and.
leave everyone to have a better idea of what Nvidia does. Because I think a lot of people talk about Nvidia and like they talk about the stock price, the earnings were this week. But really, what what is Nvidia doing? And they know that they do chips and they're related to AI. But like, what does that mean? How do they get started? Things of that nature.
So the first thing we want to talk about is the early days of Nvidia and why is it interesting? So when Nvidia was started in 1993, there wasn't this mass demand for computers. Computers were just started. I think in 1993, they asked the census and they didn't even ask whether or not the households had internet. They just asked if they had a computer. The internet question came.
in the following senses. But only 22 % of households had a computer in 1993. So this thought process of starting this company to be an integral part of computer graphics and potentially this industry called gaming at your house, it's just a really foreign concept for many people back then. And I didn't really understand because I wasn't even born yet, but.
to put it in perspective, people that weren't around before 1993, yeah, people didn't have computers at their house and you didn't have internet potentially. And people weren't gaming. You know, from what I read, it was like a big, it was a big issue of them raising funds because a lot of people were like, well, you know, we like to spend the kids spend time outside. They're not going to.
not play with their friends outside and you're expecting people to spend more time inside. And it's just like the direct opposite of what we think people are going to be doing. And so that was their pitch. They're like, we're going to start this, this company that is going to serve this industry that is still in its infancy and it will have to completely change how people live their lives. And it was very,
foreign concept for sure and definitely a tough idea to wrap your head around at that time.
But nevertheless, I mean, they succeeded, but they weren't the only ones who started research for GPUs. So there was a couple of other people that were involved, not at Nvidia, but other competitors. And during the like 2000s, there was this bust and they were one of the few companies to survive. And then after that point,
they started to research during like, I think like 2002 or something like that where they're researching to use GPUs for mathematics. And, you know, just backtrack real quick, GPUs are graphic cards. They're used for like TVs or graphics for your, you know, your computer to watch videos and.
and handle many tasks simultaneously, like generating millions of pixels on your screen. That would be something a graphics card is good at. But they also thought that graphics cards would be good at mathematics or complicated models. And so at that time, researchers were using CPUs and CPUs can handle, you know, many tasks, but
and are strong, but it's a different type of chip structure. And so it's not really designed to handle that many tasks, like billions of tasks or something like that all at once. So a graphics card, they anticipated a graphics card would be better at handling these large models that they were researching in the 2000s. And so these models that...
or were theorized in the 80s or 90s, they started trying to put together some basic mathematics models on the graphics cards. And then, lo and behold, they got a hit. They were able to...
they were able to do some cool stuff with the graphics cards. And so they saw that there was something there and that they could start using that for research. And Nvidia, after they realized this, they used the revenue that they got from the gaming. So the gaming industry was kind of propped up Nvidia and helped the company grow. But Nvidia wanted to expand further than just the gaming industry because
you know, you, you, you obviously want it, you, you want to have an industry to be your cash cow, but then once you start making money, you, you want to expand and diversify your portfolio. So that's what Nvidia was trying to do. And so Nvidia was trying to use the research and R and D that they spent on the graphics cards, excuse me, on the graphics cards to expand into.
research. But one thing that kind of happened while they were doing all this was crypto mining. So crypto mining became a big thing and it was kind of like a bubble, but people were buying up and companies were buying up these graphics cards to use them for crypto mining. And, and so basically, you know, you'd use these graphics cards cause they, they can handle all these tasks simultaneously. You would use them to do.
Um, the, what is it?
the crypto mining parameters or I don't know. You have to mine the keys and to get the key to get the keys, you have to do this hard math problem. And every time, um, sick, there is a key release, the key gets harder. And so it, it costs more resources to mine the next key and it just becomes exponentially more difficult over time. And so you use the graphics cards, but
to mine crypto, it required more and more graphics cards and it became more and more demanding resource wise for the competition needed to can successfully mine your cryptocurrency. So that happened and that was kind of, I don't know, maybe like a five year span of just graphics cards being sold out and gamers complaining.
not be able to build their rigs because it, you know, their rig is not, is, is not what it is without a Nvidia graphics card. And then in like 2012, GPUs got faster than CPUs for machine learning. And that's when Nvidia was able to kind of expand into the machine learning space and machine learning.
seemed to be something that was viable that we could be do, we could actually do. So we had the models and the concepts, but on the CPU, it wasn't really running and it wasn't as efficient. I mean, they could run it, but it wasn't as efficient as using a GPU. I'm not saying you couldn't do it, but the models that they were trying to do and the scale that they were trying to reach wasn't achievable with a CPU.
So moving over to the founders, we had three founders. We have Curtis Grimm, Chris Maliszkowski, and Jansen Yeung.
Oh, I think, sorry. I practiced the pronunciation of the names before the podcast, but during the podcast, it seems like I'm messing them up. But anyways, so Jensen, Jensen, sorry, Jensen. I'm messing up the names now. I'm just all sorts of the combined miscombobulated, but Jensen has a demanding leadership style and he's the current CEO and.
president and he has been since the inception of the company. But his leadership style is kind of interesting where he has this kind of high demand, high results atmosphere. And that's very typical of these kind of revolutionary companies, I guess. I don't know. We're going to keep rolling with it. But so for companies like this, I mean, you know, you put them in the same state as like,
Prime IBM or Prime Microsoft or you know, Prime Apple when Steve Jobs was, was part of the company and they were doing all these hard things that people have never, never experienced. And the atmosphere is pretty similar where there's high demand, you know, for, for, for results and anything besides the results is really not acceptable. But one of the, one of the cool things that,
NVIDIA is known for is promoting youth within. So when I say youth, it's not like a like a young, like a young kid, but basically they will take they'll take a big bet on a college grad or a recent college grad, maybe like two years experience, and they'll make them the head of a massive project and they'll spearhead the project.
without the experience, but as long as they're ambitious and driven and obviously smart, they would be given the opportunity to run the project and take the lead. And what the response was like kind of like the thought process behind it, NVIDIA is like, they said it was good to do that and use a young leader because they're inexperienced and that way they don't necessarily, they're not held back by.
other people's ideologies or they're just fresh. Like they're, they're just, they see what they need to get done and they just do it. And there's nothing holding them back on, on executing what they, what is demanded of them. So it seems to work out all right. And, and it's definitely a unique leadership style. I don't know of many large companies that are like taking bets on young.
college grads that just out of out of school or maybe two years professional experience and then putting them on a massive project.
But there is kind of potential innovation trade off between shipping products and innovating. I mean, I'm not saying Nvidia isn't innovating. They're still industry leaders, but there are some criticisms, like if you're constantly focused on shipping and shipping and improving a chip, then you might be missing big pictures.
But so Yen -sen Yen -sen is, you know, the CEO and president of Nvidia. His early life was he was born in 1963 in Taiwan at the age of nine. He moved over to live with his uncle in Washington. And during that time in this and this might be part of the reason why that he. Thinks and leads the company and has that initiative to to promote.
young leaders to handle large projects is when he was in high school, he skipped two years of high school and then went to college at 16. And so that might affect like his perspective. Like, you know, if you're young, it doesn't matter. You could just get it done. But while he was graduating and after he graduated, he or why was graduating, he graduated from Oregon and then later on got his master's.
at Stanford. And then he then he obviously became a professional. He worked at AMD for a little bit and some other companies. And then at 30, he made the jump to found a video. His plan three, he really focused on or focuses on donating to Stanford in Oregon. So he donated, I think, 30 or so million to Stanford to build an additional
engineering school and then for Oregon he donated to build a supercomputer complex camp and I complex you know a complex I mean like building slash like center.
And then Curtis Ream, he retired in the early 2000s and I couldn't find much of him on the Internet. He lives off the grid, apparently. And from what I can tell from his Internet presence, I think that's true because it's super difficult to find any information about him. But before he went to Nvidia, he worked at IBM. And then Chris Maliszkowski worked at.
IBM obviously is one of the founders, but he still works there. That's what I meant to say. He works there as an executive staff assistant, like technical staff assistant of executive. And he grew up in New Jersey. So those are the founders. There's a the other two founders. There's not as much information, obviously, because they have more of a supporting role.
Whereas Jensen is the spotlight and all the news media is on him and investigation is on him. He's the CEO and president and he's obviously more involved on the media aspect. But for the next part, Core CompC's and products. So Core CompC's is the hardware that Nvidia creates. One of the things that...
Nvidia does a little bit differently than a lot of companies. And it's coming more of a common practice. I think that Samsung is doing it. Apple has definitely been doing it for a little bit. Google is starting to try to do it, but their applications are different, like Google and Apple and Samsung. I would say let's just talk about Apple because Apple for sure is doing it at a in a.
in a high capacity and consistently for a long time where they not too long, but since 2020. So good amount of time. Twenty one. Apple has been developing their silicon and their products in -house, blister software. So what the issue of the Apple was having was they.
have to, they were outsourcing their chips. So say that Intel makes the chip for the Apple phone, the iPhone, and then the chip will have certain specifications that it can't do or can do. And then the developers with Apple, when they're developing the OS, they have to.
adhere to the chip instead of the chip adhering to the software per se, where if you build your own hardware, your own software, and then obviously your own silicon, then when you're fully integrated, you don't have to abide by these other limitations. You can just do whatever you want. Obviously within reason, but.
That's kind of the idea. And so, NVIDIA does the same thing where they manufacture and design their chips in -house. Where as most competitors don't do that because it's really expensive to manufacture and design. One of the things with the manufacturing and creating these fabs is it's gotta be like this crazy contained, very expensive.
manufacturing plant that has to have insane controls for any dust particle in the air.
it completely shuts down the plant. If there's any like extra materials in the air or on the ground or anywhere, it completely shuts down the plant. And there's these crazy controls on the plant because if anything like that gets on a chip, the chip is broken. So it's very expensive to manufacture chips even to get started. So there's this large upfront cost to...
to create these plants and then to get them to be running at full capacity normally takes a good amount of time because of just training the staff and.
getting everyone used to the procedures and getting everything running like a world -old machine, it takes some time. And then also to be designing on top of that, it allows them to integrate or not integrate, but innovate faster than other people. And it gives them more control, but also gives them some supply chain, potential supply chain issues. And that happened during COVID.
and people, they couldn't get chips. And if they were trying to get chips, they were super duper expensive. And I think I'm pretty sure that Tesla used to be very part like a close partnership with Nvidia for their self -driving. And I'm pretty sure also Nvidia, it provides the drive platform to Waymo, which is the Google self -driving startup.
I don't know if it's a startup, if it's backed by Google, but whatever. But, so.
when they had the supply issues, NVIDIA was providing the chips that were reused to train the AI. And then they were also providing for Waymo, they were providing the software, the drive platform. So what happened was it really messed up Tesla because Tesla was relying on these NVIDIA chips for their Teslas. And so that prompted Tesla to build their own chips.
And so when they were fully integrated like that vertically, it did cause some issues supply chain wise.
And so the core competencies, you know, hardware, and then they also have, you know, AI models. They, they not only help run the AI models, but they also develop their own AI models for things like, you know, language processing and image generation. So not only do, are they fully integrated on the, on this hardware side, but they also, they're providing software that, you know, developers can use or, or use to research.
for various reasons. And then for talent in R &D, talent, they have a reputation of poaching from top universities with very aggressive salaries, definitely at the top level. And, you know, they also have a reputation of demanding very long hours to...
their employees so that might drive off people. But that also, it only allows pretty much for people that are ambitious and want to ship these crazy ideas and challenge themselves. So it's kind of a double edged sword. I think, you know, you're encouraging people that that want to get stuff done and people that just want to. And there's nothing wrong with that. There's nothing wrong with just wanting to have a nice.
work -life balance. But if you don't want to work long hours, you probably don't want to work at Nvidia. So that's why I'm saying it's a double -edged sword. R &D, they spend a crazy amount of percentage of their revenue on R &D versus others in the sector. And, you know, they're, one example is like they're one of their recent chips to H100 GPU has like,
80 billion transistors, which is like 68 % more than the previous generation. And so their innovations on chip to chip is, you know, extensive.
So core products. So I wanted to take some time to explain what architectures are and give some examples. And then, you know, what is their enterprise and developer platform? Like, you know, we'll give an example of a platform that people use, the gaming and industry technologies. So that's basically something I ripped off their site where they go to products, their staff sectors for each of their products, categories.
And then within those categories, they have like 20 offerings. So definitely not going to go over all of them. I'm just going to list one of them, give an example, list one of them, give an example, just so you have a better idea. So architectures. So there, you know, there's the, the Ada and then Love Lance. So Love Lance and Ada that could be used for ray tracing. So for a game like Fozor, Fozor, Fozor, Fozor motorsport, it allows.
for the rate tracing, which basically enables photo -ristic video graphics, which I've played for it, and the graphics are incredible. So thank you, NVIDIA, I appreciate you. Enterprise and Developer. So there is a platform called Omniverse, and that's a platform that's deployed.
by Nvidia and an example that I have obviously is played by Nvidia but the example I have is Lockheed Martin uses Omniverse to create a digital twin of the earth to model climate change for climate change research gaming. So we have the GeForce RTX which is like the gold standard, the graphics card. It's the
graphics card that pushes the boundary of gaming and allows for hyper realistic videos and simulations, which is really great. And once again, appreciate Nvidia for letting us gamers game. And so industry technologies, I talked about earlier, but they provide a platform called Drive. And so Drive is something that allows them to...
integrate with the hardware that that Nvidia provides to process the data in real time.
So those are some examples, but how is it made or what is it? What is an architecture? So an architecture is kind of like a, let's say like a blueprint. So it's like a blueprint of these different components that allow for different workflows. So you can have an architecture for, I don't know, you can have an architecture for gaming, you can have an architecture for machine learning that a data science would use.
And so these different architectures are these different Nvidia cards for different purposes. And that's basically what an architecture is. There's a turning architecture, there's the Ampere architecture. There's a whole bunch, I can't name all of them, but that will give you a general idea of what an architecture does and just think about it as like a blueprint of the chip and what the use case is gonna be. That's what the architecture is. A CPU is kind of like,
I would describe it as the brain of the computer. And so it's good at running, you know, programs or general tasks. And then a CPU is, or not CPU, a GPU is the graphics. You know, this, this is like the power house that can, that can run these massive parallel tasks, um, like rendering like millions of pixels at once or for gaming. This is what I, example I said earlier or
you know, for AI applications.
Semiconductor is kind of like this like a catch -all term and its primary material is silicon. And it's built to control and block electricity and it controls the flow of electricity, long story short. So how are these things built? So this is a super simple.
overview, it's like four steps. It's definitely not this simple. It's definitely way more complicated. But for the essence of this discussion, let's just make it simple. Because even I have some abilities. So I would start, you start with like sand and then so you apparently you refine down sand to these crazy purity levels. And then,
from the sand, you do the wafers, which is these like super thin disks in a clean room environment. And I said earlier in the conversation that you can't have any dust in the air and it has to be very sterile. If there is a dust, it ruins the chip. And a photo with graph key, I think is how you pronounce it, but they, you know.
put the stencil of the wafer using light and then you, I guess you etch away parts of it using chemicals. I'm not so sure. And then, and then from this, from this step, you would create these patterns on the chip that you would see when you look at a chip, you see all this little patterns. That's, that's what that is. And then there's many hundreds of other steps, but in a grand scheme of things, that's
what and how CPUs and GPUs are created. And there's many other steps, once again, and that's why it's super expensive because if there's anything that gets messed up in these hundreds of steps, it's ruined. So, I don't know, NVIDIA is something else. One thing that I didn't say and I thought was pretty cool was NVIDIA's latest chip has more transistors.
than the neurons in the human brain. That's pretty cool. I think, think Nvidia is more than these shiny graphics cards. They're pushing out these AI tools and putting them in the hands of creators and scientists. And they're changing the way that we think about the biggest problems, the world's biggest problems. And I don't know, I think that more people should be interested in, and not only interested, but,
knowledgeable about these companies and what they're doing, not only their stock price, but you know how they're doing it, their story. I find it interesting and I appreciate you diving in with me today with Nvidia. I hope you learned something new and if you'd like to watch this on the video or share it or listen to it with your friends, you know, you can listen to it on Apple.
Spotify, Spotify, Spotify and YouTube and so and so, but those are the main ones. If you would like to watch it via video, that would be on YouTube and, or I guess YouTube music. I'm kind of confused about this whole Google thing as well. Uh, but you could also watch the video on Spotify, upload an audio in a video version just so you can have it. Appreciate it. See you next week. Have a great day. Bye.
Creators and Guests
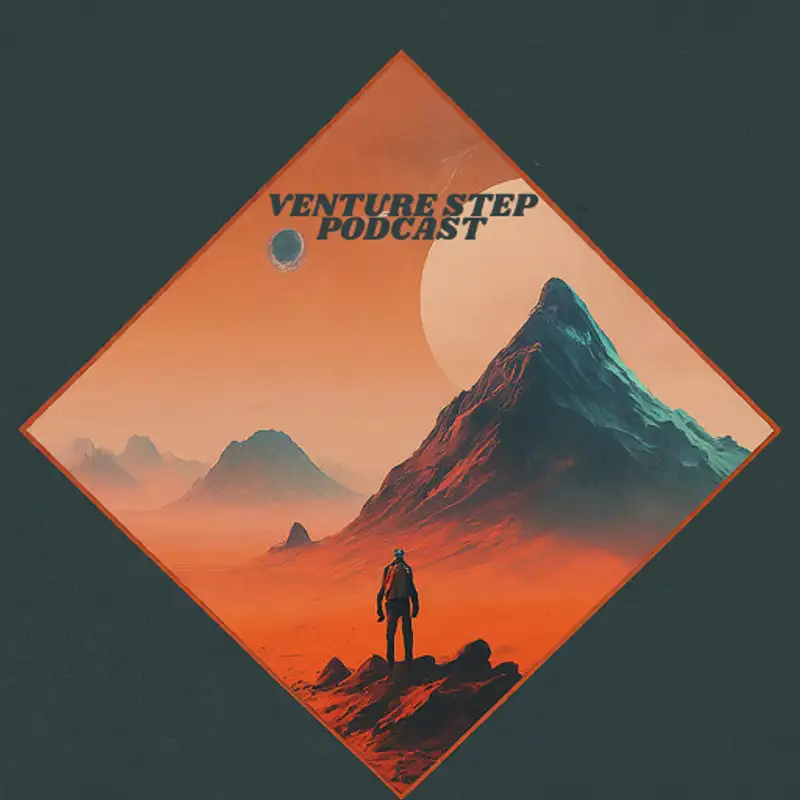